The Power of Predictive Analytics
Smart universities are turning to new data sources to identify those students who need a nudge toward success.
- By Dian Schaffhauser
- 09/25/13
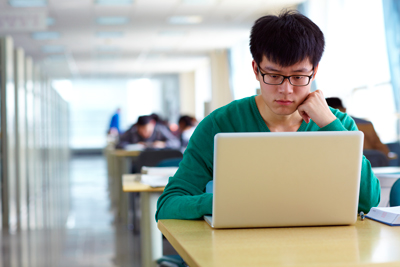
Photo: iStockphoto |
The promise of predictive analytics in higher ed continues to entice--for good reason. It can change student lives. In the two years that the American Public University System has been applying predictive analytics to its online learners, for example, the dropout rate has fallen by 17 percent. To achieve this result, APUS analyzes 187 data points that help pinpoint students who are likely to withdraw within the next five days. According to Phil Ice, APUS' VP of research and development, this capability "has catalyzed a whole series of changes in the way that faculty and advisers interact with students."
So why aren't more schools taking a similar approach? For one, most institutions are struggling simply to leverage the traditional data points they gather about students, let alone 187 often-arcane ones. Second, a data mix that works at one school may not be relevant at another, making it almost impossible to buy an effective packaged solution off the shelf. Finally, the creepiness factor needs to be resolved: Just how intrusive can schools be in tracking the actions of their students?
Misplaced Priorities
And the elephant in the room? Schools are mining the wrong data. At most schools, the money dedicated to data collection, analysis, and reporting goes into areas with little direct impact on student success. "[Institutional research] departments spend the majority of their time on accreditation, licensure, board reporting, and even Freedom of Information requests," complains Mark Milliron, founding chancellor of Western Governors University Texas and new chief learning officer for Civitas Learning, an education analytics startup. "But I fundamentally believe that the power of data doesn't get unlocked until you get the data to the front lines." He wants to see some of the research money used "to give students and faculty access to information so they can do a better job."
In Milliron's view, the situation is dire. When he taught in community colleges and universities, he says, the only piece of student information he typically received was a class list--and even that was often incomplete. Compare his experience to that of another group on campus: sports coaches. "Every single coach at the University of Texas at Austin has a full workup on every single athlete they're going to work with next year--strengths, weaknesses, all the background," notes Milliron. "And they're actually going to develop a plan for that player from point A to point B. I don't begrudge that of the coaches; I just think it would be great for the faculty to have that stuff, too."
But Milliron cautions that achieving success with predictive analytics isn't easy. Some people overreach--overselling its power and underselling how complex the work is--while others rebel against making changes based on what the data shows.
The Drive to Big Data
It can also be difficult for a single school--particularly a small one--to develop data sets large enough to be statistically valid. As a result, any analysis based on a small data set--even if it eventually turns out to be spot-on--is likely to spur some doubt.
This was certainly the experience of Ice when he joined APUS five years ago, even though the school has a large enrollment of more than 100,000 students. According to Ice, he and his team "built some very good models around why students were dropping out," but some of their findings seemed extraordinary.
First, students who had transfer credit--even a single credit--were four times more likely to stay enrolled than students who arrived without any credits. Second, neither ethnicity nor gender were significant predictors of whether a student would drop out. "That is, in itself, absolutely stunning," notes Ice. Until then, he explains, research had concluded that minorities were less likely to succeed than Caucasians. APUS' data said otherwise. "Online learning is totally color-blind," says Ice. "The non-significance of that finding was probably one of the most significant things we ever found."
Because APUS' findings ran counter to the prevailing research, Ice initially doubted his own analysis. He wanted a reality check, a way to compare his team's findings with those from other schools. This desire for corroboration prompted the school to join the Predictive Analytics Reporting (PAR) Framework, with Ice as the principal investigator. PAR is an initiative by the Western Interstate Commission for Higher Education Cooperative for Educational Technologies (WCET) that, according to its website, "brings together two-year, four-year, public, proprietary, traditional, and progressive institutions to collaborate on identifying points of student loss and to find effective practices that improve student retention."
With 16 institutions participating, PAR is now able to sift the anonymized records of 1.6 million students as well as 8.1 million course-level records. As for APUS' finding about ethnicity, another PAR pioneer, Rio Salado College (AZ), reached the same conclusion.
Mining Trace Data
Learning management systems are an obvious source of data about learning, which explains why so many LMSes now feature analytics modules. The LMS is also where many schools have focused their analytics work, assuming that the "sweet spot" for gauging student engagement lies in how quickly or frequently students participate in class activities.
But limiting your school's focus to the LMS is a big mistake, according to Rey Junco, an associate professor of library science at Purdue University (IN) and a faculty associate at the Berkman Center for Internet & Society at Harvard University (MA). Junco believes the current state of LMS research is too basic. "We're just focusing on the number of posts on an LMS, or the number of times a student logs in," he complains. The problem with these kinds of data points, he says, is that often what are "being looked at in terms of prediction are also actually course requirements. Of course the number of posts on the course-management system predicts success--the faculty member is grading the student on the posts."
Junco encourages schools to cast a wider net. "There's so much more data that can be collected," he notes. "We're really missing out." He has coined the term "trace data" to describe the "bits of data that students leave behind." It's valuable because it can be used as "proxies for all sorts of variables...related to student success."
Examples include such "throwaway" data as card swipes that show how often students use a library resource, or what activities they perform on their computers and for how long. Or it might be how long students spend in their digital textbooks, a project for which Junco is acting as an adviser for digital publisher CourseSmart. "It's so incredibly innovative to track textbook usage," he proclaims. "Heck, why didn't we think of this before?"
E-book Data
The CourseSmart initiative provides faculty members with an analysis of student usage of the company's e-texts. Each time a student views a page in an e-text, the detail is recorded along with session length and other data points. The data is then compiled into an online report that faculty can view either on a classwide basis or by individual student. What transports the CourseSmart data into the realm of predictive analytics is its use of an "engagement index," a number that gauges how engaged a student is with the material.
Adrian Guardia, an instructor in the College of Business at Texas A&M University-San Antonio, monitored three undergraduate classes--one online and two hybrids--as part of a pilot to test CourseSmart Analytics. About four weeks into the spring semester, he identified three students in the online class who hadn't done well on quizzes and who also had low engagement index scores. He reached out to all three, supplying them with copies of their analytics reports, and two responded.
"They were surprised that I made the effort to contact them," Guardia recalls, noting that his interaction with the students sparked conversations about the obstacles in their lives that stood in the way of schoolwork. In the end, all three students (even the one who didn't meet with him) pulled up their engagement indexes, signaling that they were hitting the books. "I think our connection inspired them to reassess their habits and reaffirm their commitments to their studies," Guardia concludes. And nobody in the pilot failed the class--an unusual event, in his experience.
While these success stories sold Guardia on the value of the CourseSmart data, it also caused him to reevaluate some of his own instructional materials. By reviewing the engagement index, Guardia discovered that even top-performing students were less engaged in the textbooks than he would have predicted. "I had spent a good amount of time and effort in selecting books that were up-to-date," he notes. "They were interesting and entertaining. They had color pictures, interesting stories, games, PowerPoint slides, videos." In spite of these multimedia riches, engagement indexes for even the best students came in around 40, not the 60 or 70 Guardia would have predicted. (The index scale runs from a low of 20 to a high of 80.)
"It really caught me off guard," he recalls. "I didn't know what to make of it." Now he's on a quest to figure out how to incorporate the e-texts into some of his course assessments in a bid to spur more engagement with the materials. One idea: open-book quizzes developed from content in the e-texts. If these new strategies don't have the desired impact, he may ultimately decide to replace the e-text with other resources.
Privacy Matters
Naturally, all these initiatives assume that students won't mind having their traces vacuumed up and sifted for particles of insight. And, for the most part, it appears they don't care. According to Cindy Clarke, CourseSmart's senior VP of marketing, 75 percent of surveyed students told the company they were comfortable having their behavior tracked. The remainder were either indifferent or not opposed. Nevertheless, CourseSmart does provide an opt-out on its website and follows the practices laid out by TRUSTe, which certifies sites and services on their privacy practices.
It's quite possible, though, that student acceptance of this data gathering stems from pure obliviousness. Through his participation in the Berkman Center, Junco is part of a Microsoft-sponsored group that is studying student privacy. When it comes to "spooky predictive models," he says, there's a general lack of awareness among the public about how they work and what they do. "If you ask most people, 'Why do you think you're seeing this ad?' and point to it on their computer screen, they're going to say, 'I don't know.' There's a lack of understanding about how these things work as we use them in our day-to-day lives."
Junco recommends that schools openly share how they collect data about their students and how it could be used. "Hopefully, talking about the educational data can help students transfer that knowledge and skills to the rest of their online world," he says.
Wanted: Data Jockeys
As far as APUS' Ice is concerned, the sky's the limit when it comes to the potential of predictive analytics in higher education. The next big thing at his institution is repurposing software from Adobe Marketing Cloud (previously Omniture), which delivers personalized experiences online. In a business setting, for example, the tool might make purchasing recommendations based on analytics about what the customer is currently doing. "I'm not saying that education is a commercial transaction," says Ice, "but the math that underlies how to get someone to a point of sale can be repurposed to understand what type of content and educational experience to provide to a student to optimize learning outcomes."
The biggest barrier to this bright future, says Ice, is finding qualified people who understand the software and the complex math involved. "Higher ed is so short of the human capital they need around analytics," he laments. "A lot of [institutions] are not going to be able to implement the extremely robust stuff by themselves."
The hope is that organizations like WCET's PAR Framework and companies like Civitas can fill the gap, bringing together schools to share the work of compiling and normalizing the data, creating models, and customizing them for individual use. But Ellen Wagner, executive director of WCET, cautions against looking too far ahead. In her view, most schools aren't ready to start doing "all kinds of unstructured, deep-dive, Hadoop types of analysis to find patterns we've never seen before." Better, she suggests, to "find insights from the data we already collect."
Wagner's prediction? The next few years will be a "really interesting time of trying things out and looking for answers. I think everyone is a little nervous."
3 Predictions for Analytics
1) Multiple Learning Paths. Phil Ice, VP of research and development at the American Public University System, believes that learning analytics will drive development of courses that provide multiple learning paths. As a result, he says, "you're going to have to build out six to seven times more content for one course than you do now." This will jack up the cost of developing and maintaining a single course by as much as $100,000. To share the burden, says Ice, universities will begin to partner in the creation of a common curriculum for 100-level classes. At that point, he adds, "the real differentiator becomes the faculty members at each institution--how they add their personal touch and the interactions [they have] with the students."
2) Assessments Based on Trace Data. Rey Junco, a faculty associate at the Berkman Center for Internet & Society at Harvard University (MA), sees great promise in the growing use of trace data for creating assessments. He compares it to the Minnesota Multiphasic Personality Inventory, a personality test with hundreds of questions that provide insight into a person's mental health. "They use statistics alone to be able to discriminate between groups of people who have certain psychological disorders," says Junco. "So a question that looks as if it [is completely unrelated] could tell you if a person is depressed, for instance, or if a person is lying about their answers." Junco believes trace data could be used in similar fashion. "There are going to be variables in the cloud [of trace data] that are going to be highly predictive of students' behavior and characteristics that lead to their success or lack thereof."
3) Instruction Based on Learning Styles. Mark Milliron, chief learning officer of Civitas Learning, foresees the day when students will be able to use an app that guides them to the most effective learning resources based on how they learn best. "[It might say] something simple like, 'A student similar to you who was stuck on this chemistry concept found these three learning objects to be useful. Click here to use them.' Wouldn't that be great?" he marvels. "That's not rocket science. This is stuff we can do if we can connect the dots."
|