MIT Researchers Aim to Bring Neural Networks to Smartphones
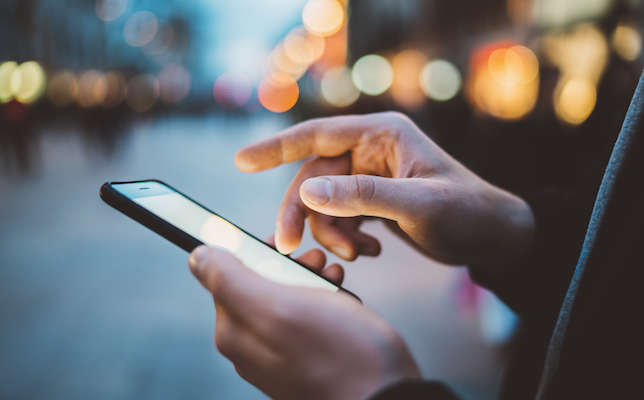
A team of researchers at the Massachusetts Institute of Technology is working to bring neural networks to handheld devices.
Neural networks comprise thousands or millions of simple and densely interconnected nodes that are organized by layers. Each interconnection has a weight and those weights are continually readjusted as the network is changed.
All that computation requires a lot of memory and power, so neural networks are better suited to run on servers than smartphones.
But last year a team led by Vivienne Sze, an associate professor of electrical engineering and computer science at MIT, developed an energy-efficient chip designed for neural networks that might allow them to run on smartphones.
"Now, Sze and her colleagues have approached the same problem from the opposite direction, with a battery of techniques for designing more energy-efficient neural networks. First, they developed an analytic method that can determine how much power a neural network will consume when run on a particular type of hardware. Then they used the method to evaluate new techniques for paring down neural networks so that they'll run more efficiently on handheld devices."
In a paper to be presented at the Computer Vision and Pattern Recognition Conference this week, the team claims that its methods reduce power consumption up to 73 percent compared to standard implementations and up to 43 percent over the previous best methods for reducing power consumption.
"The first thing we did was develop an energy-modeling tool that accounts for data movement, transactions and data flow," Sze explained in an MIT report about her team's research. "If you give it a network architecture and the value of its weights, it will tell you how much energy this neural network will take. One of the questions that people had is 'Is it more energy efficient to have a shallow network and more weights or a deeper network with fewer weights?' This tool gives us better intuition as to where the energy is going, so that an algorithm designer could have a better understanding and use this as feedback. The second thing we did is that, now that we know where the energy is actually going, we started to use this model to drive our design of energy-efficient neural networks."
One method for reducing the energy consumption of the networks is called pruning, or the elimination of low-weight connections, which tend not to affect the eventual output of neural networks.
Pairing their energy model with this technique, Sze and her team cut away the network layers that consume the most energy using a method they dubbed "energy-aware pruning."
The weights between connections can also be positive or negative, so the team looked for connections that cancel each other out and eliminates them as well.
"The inputs to a given node are the outputs of nodes in the layer below, multiplied by the weights of their connections," according to an MIT report about the research. "So the researchers' method looks not only at the weights but also at the way the associated nodes handle training data. Only if groups of connections with positive and negative weights consistently offset each other can they be safely cut. This leads to more efficient networks with fewer connections than earlier pruning methods did."
More information about neural networks is available at news.mit.edu.
About the Author
Joshua Bolkan is contributing editor for Campus Technology, THE Journal and STEAM Universe. He can be reached at [email protected].